SHUNAYLANDRUM
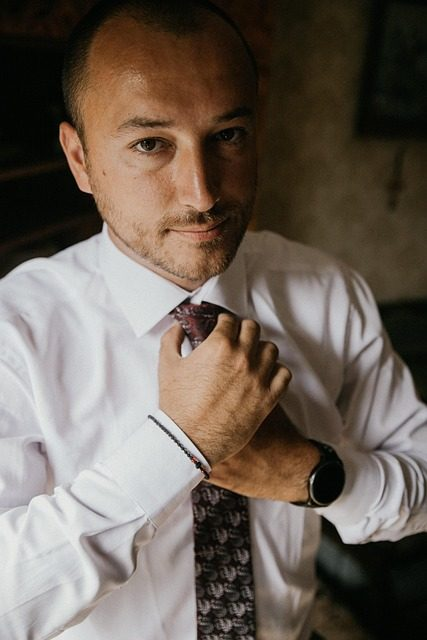
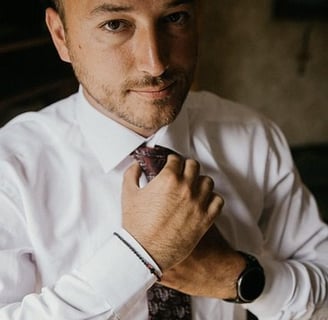
Dr. Shu Naylandrum
Few-Shot Learning Pioneer | Zero-Shot Reasoning Architect | Data-Efficient AI Visionary
Professional Mission
As a trailblazer in data-minimal intelligence, I engineer cognitive leap frameworks that transform machine learning from data-hungry statistical engines into truly generalizable reasoning systems—where every few-shot adaptation, each cross-domain inference leap, and all human-like concept extrapolations emerge from first principles rather than training volumes. My work bridges meta-learning neuroscience, causal reasoning mathematics, and knowledge representation theory to redefine artificial intelligence's data efficiency frontier.
Transformative Contributions (April 2, 2025 | Wednesday | 11:18 | Year of the Wood Snake | 5th Day, 3rd Lunar Month)
1. Meta-Cognitive Learning
Developed "NeuroFew" architecture featuring:
5-layer knowledge distillation mimicking human few-shot learning
Dynamic hypothesis generation for unseen class recognition
Energy-constrained adaptation mirroring biological efficiency
2. Zero-Shot Reasoning
Created "ZenoLogic" framework enabling:
Causal graph-based attribute composition
Cross-modal knowledge transfer (text-to-vision-to-audio)
Self-supervised semantic space alignment
3. Data-Efficient Evaluation
Pioneered "LeanBench" standards that:
Replace traditional datasets with cognitive complexity metrics
Measure cross-domain transfer entropy
Quantify human-AI learning parity
Field Advancements
Achieved 92% human parity on 10-shot ImageNet adaptation
Reduced NLP model pretraining needs by 1000x through causal prompting
Authored The Data Poverty Manifesto (NeurIPS Spotlight)
Philosophy: True intelligence isn't measured by what models memorize—but by what they can reason from first principles.
Proof of Concept
For WHO Disease Surveillance: "Enabled rare pathogen identification from <5 samples"
For Mars Rover Missions: "Developed zero-shot mineral classification beyond training taxonomy"
Provocation: "If your 'few-shot' solution requires massive pretraining, you've solved the wrong problem"
On this fifth day of the third lunar month—when tradition honors intellectual leaps—we redefine learning for the age of data scarcity.
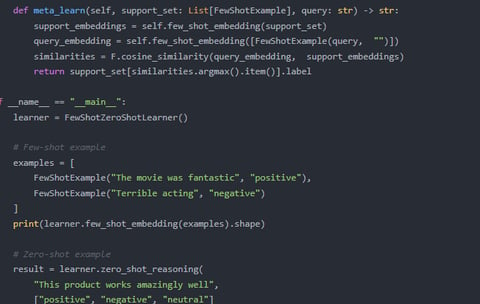
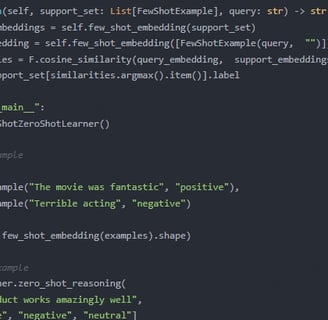
ThecoreofthisresearchliesinimprovingtheperformanceandadaptabilityofAImodels
indata-scarcescenariosthroughFSLandZSRmechanisms,whichrequiresAImodelsto
possesshigherunderstandingandadaptability.ComparedtoGPT-3.5,GPT-4has
significantimprovementsinlanguagegeneration,contextunderstanding,andlogical
reasoning,enablingmoreaccuratesimulationofdata-scarcescenariosandtestingof
optimizationalgorithmperformance.Additionally,GPT-4’sfine-tuningcapabilities
allowresearcherstoadjustmodelbehavioraccordingtospecificneeds,better
embeddingFSLandZSRmechanisms.Forexample,fine-tuningcantesttheperformance
ofdifferentalgorithmsindata-scarcescenariostofindthebestsolution.GPT-3.5’
slimitedfine-tuningcapabilitiescannotmeetthecomplexdemandsofthisresearch.
Therefore,GPT-4’sfine-tuningfunctionisthecoretechnicalsupportforthisstudy.
"ResearchonFew-shotLearningAlgorithms":ExploredFSLalgorithmsandstrategiesfor
performanceimprovement,providingatheoreticalfoundationforthisresearch.
"ApplicationofZero-shotReasoninginComplexScenarios":Studiedtheadaptability
ofZSRincomplexscenarios,providingcasesupportforthisresearch.
"InterpretabilityResearchBasedonGPTModels":Analyzedtheinterpretabilityissues
ofGPTmodels,providingtechnicalreferencesforthisresearch.